Elasticsearch: Working With Dynamic Schemas the Right Way
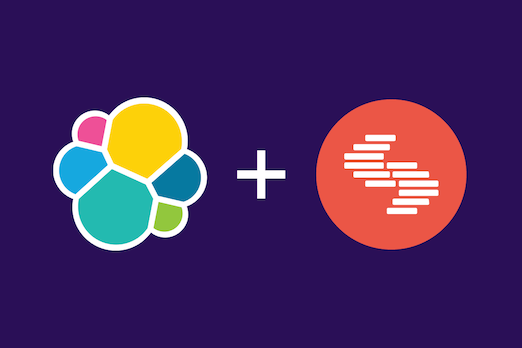
Elasticsearch is an incredibly powerful search engine. However, to fully utilize its strength, it’s important to get the mapping of documents right. In Elasticsearch, mapping refers to the process of defining how the documents, along with their fields, are stored and indexed.
This article dives into the two types of schemas (strict and dynamic) that you usually encounter when dealing with different types of documents. Additionally, we look at some common but useful best practices for working with the dynamic schema so that you get accurate results for even the most complex queries.
If you are new to Elasticsearch, we recommend reading and understanding the related terms and concepts before starting.
Schema Types, Their Mapping, and Best Practices
Depending on the type of application that you are using Elasticsearch for, the documents could have a strict schema or a dynamic schema. Let’s look at the definition and examples of each, and learn more about their mapping.
Strict Schema - The Simple Way
A strict schema is where the schema follows a rigid format, with a predefined set of fields and their respective data types. For example, systems like logs, analytics, application performance systems (APMs), etc. have strict schema formats.
With such schemas, you know that all the index documents have a known data structure, which makes it easier to load the data in Elasticsearch and get accurate results for queries.
Let’s look at an example to understand it better. The following snippet shows the data of a log entry within Nginx.
{ "date": "2019-01-01T12:10:30Z", "method": "POST", "user_agent": "Postman", "status": 201, "client_ip": "0.0.0.0", "url": "/api/users" }
All the log entries within Nginx use the same data structure. The fields and data types are known so it becomes easy to add these specific fields to Elasticsearch, as shown below.
{ "mappings": { "properties": { "date": { "type": "date" }, "method": { "type": "keyword" }, "user_agent": { "type": "text" }, "status": { "type": "long" }, "client_ip": { "type": "IP" }, "url": { "type": "text" } } } }
Defining the fields, as shown above, makes it easy for Elasticsearch to get the relevant results for any query.
Non-Strict Schema Challenges and How to Overcome Them
There are several applications where the schema of the documents is not fixed and varies a lot. An apt example would be the various structures that you define in a content management system (CMS). Different types of pages (for example navigation, home page, products) may have different fields and data types.
In such cases, if you don’t provide any mapping specifications, Elasticsearch has the ability to identify new fields and generate mapping dynamically. While this, in general, is a great ability, it may often lead to unexpected results.
Here’s why:
When documents have a nested JSON schema, Elasticsearch’s dynamic mapping does not identify inner objects. It flattens the hierarchical objects into a single list of field and value pairs. So, for example, if the document has the following data:
{ "group" : "participants", "user" : [ { "first" : "John", "last" : "Doe" }, { "first" : "Rosy", "last" : "Woods" } ] }
In such a case, the relation between “Rosy” and “Woods” is lost. And for a query that requests for “Rosy AND Woods,” it will actually throw a result, which, in reality, does not exist.
So, What’s the Solution to This?
The best way to avoid such flat storage and inaccurate query results is to use nested data type for fields. The nested type is a specialised version of the object data type that allows arrays of objects to be indexed in a way that they can be queried independently of each other.
This makes sure that the relation between the objects, if any, is maintained, and the query would return accurate results. The following example shows how you can add a generic schema for all pages of a CMS application.
{ "mappings": { "properties": { "doc_type": { "type": "keyword" }, "doc_id": { "type": "long" }, "fields": { "type": "nested", // important data type "properties": { "field_uid": { "type": "keyword" }, "value": { "type": "text", “fields”: { “raw”: { “type”: “keyword” } } } } } } } }
Now let’s look at a couple of examples where different types of input objects can be ingested into a single type of index.
Example data 1:
{ "first_name": "ABC", "last_name": "BCD", "city": "XYZ", "address": "Flat no 1, Dummy Apartment, Nearest landmark", "country": "India" }
You can convert this data into Elasticsearch mapping, as shown below:
{ "doc_type": "user", "doc_id": 500001, "fields": [{ "field_uid": "first_name", "value": "ABC" },{ "field_uid": "last_name", "value": "BCD" },{ "field_uid": "city", "value": "XYZ" },{ "field_uid": "address", "value": "Flat no 1, Dummy Apartment, Nearest landmark" },{ "field_uid": "country", "value": "India" }] }
Example data 2:
{ "title": "ABC Product", "product_code": "PRODUC_001", "description": "Above product description colors, sizes and prices", "SKU": "123123123123", "colors": ["a", "b", "c"], "category": "travel" } { "doc_type": "product", "doc_id": 100001, "fields": [{ "field_uid": "title", "value": "ABC Product" },{ "field_uid": "product_code", "value": "PRODUC_001" },{ "field_uid": "description", "value": "Above product description colors, sizes and prices" },{ "field_uid": "SKU", "value": "123123123123" },{ "field_uid": "colors", "value": ["a", "b", "c"] },{ "field_uid": "category", "value": "travel" }] }
This type of mapping makes it easier to perform a search on multiple types of documents within an index. For example, let’s try to search for users where "country" is set to "India" AND for products where "category" is set to "travel."
GET /{{INDEX_NAME}}/search { "query": { "nested": { "path": "fields", "query": { "bool": { "should": [ { "bool": { "must": [ { "match": { "fields.field_uid": "country" } }, { "match": { "fields.value": "India" } } ] } }, { "bool": { "must": [ { "match": { "fields.field_uid": "category" } }, { "match": { "fields.value": "travel" } } ] } } ] } } } } }
In Conclusion
If you are certain that your documents follow a strict schema, you don’t need to structure your data in a nested data type format. Follow the pattern shown in the “Strict Schema” section to input your data in Elasticsearch.
However, suppose your documents are not likely to follow a strict schema. In that case, we highly recommended that you store the data in a nested format, which helps you consolidate all types of documents under a single index roof with uniform mapping.
About Contentstack
The Contentstack team comprises highly skilled professionals specializing in product marketing, customer acquisition and retention, and digital marketing strategy. With extensive experience holding senior positions in notable technology companies across various sectors, they bring diverse backgrounds and deep industry knowledge to deliver impactful solutions.
Contentstack stands out in the composable DXP and Headless CMS markets with an impressive track record of 87 G2 user awards, 6 analyst recognitions, and 3 industry accolades, showcasing its robust market presence and user satisfaction.
Check out our case studies to see why industry-leading companies trust Contentstack.
Experience the power of Contentstack's award-winning platform by scheduling a demo, starting a free trial, or joining a small group demo today.
Follow Contentstack on Linkedin
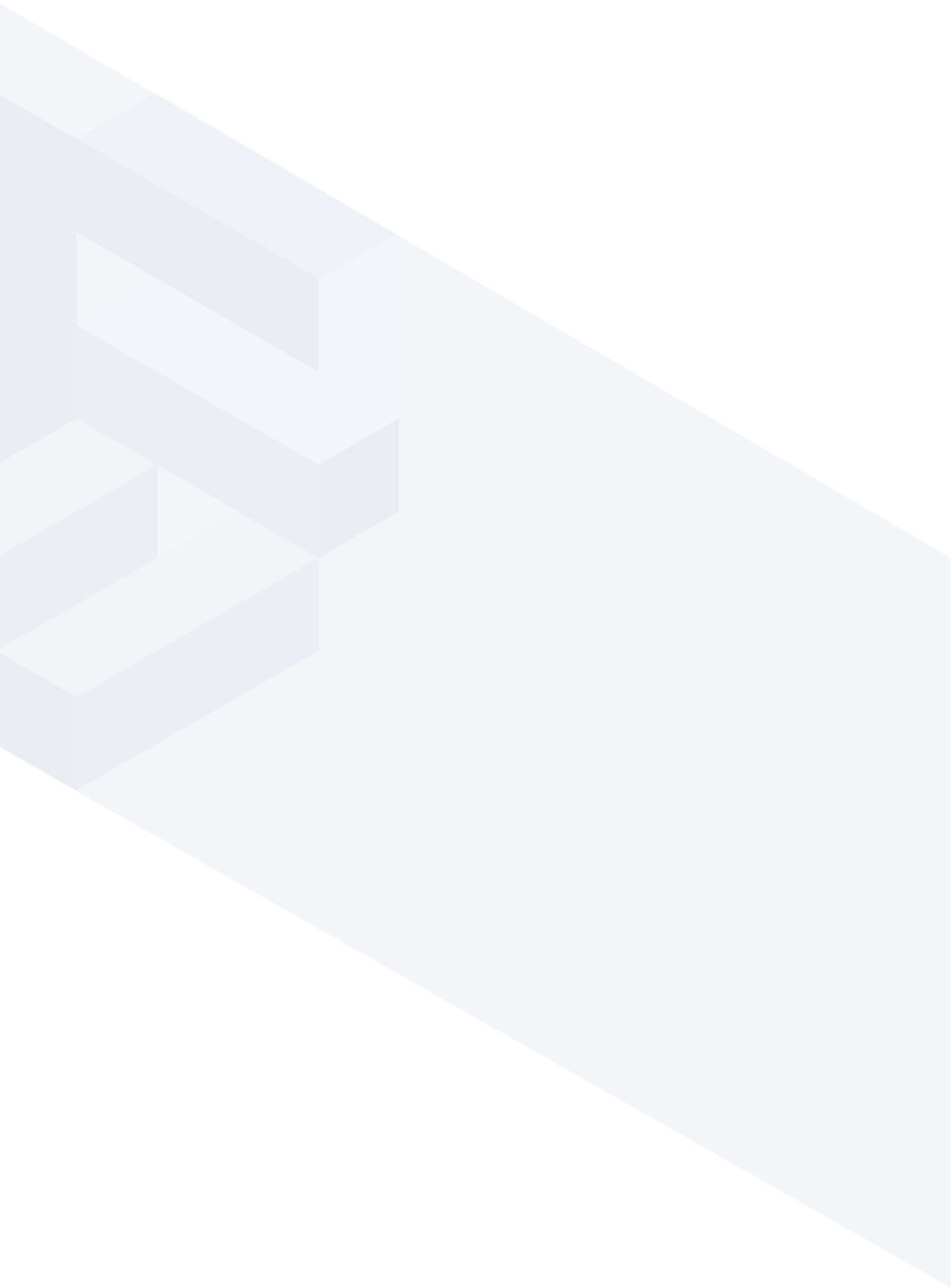