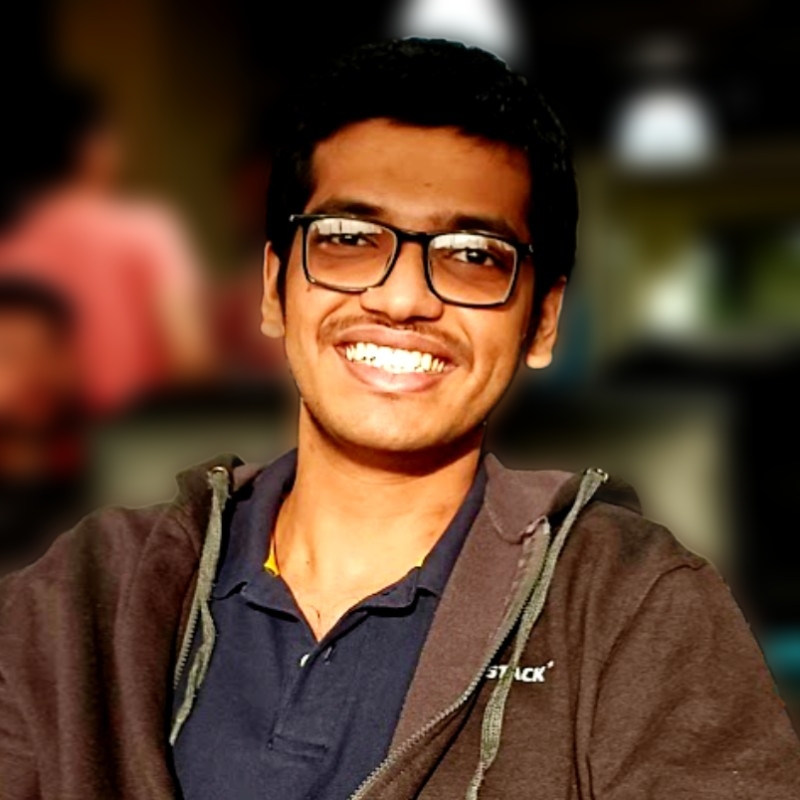
Vivek Singh
Vivek Singh, a Machine Learning Engineer, enjoys crafting scalable solutions for customers infused with machine learning. He's an avid reader of tech blogs and spends his free time creating small proof-of-concepts. You might also find him watching or playing football.